OAS to Tool Schema
OpenAPI Specification is a standardized format used to describe and document RESTful APIs. The OAS format enables the generation of both human-readable and machine-readable API documentation, making it easier for developers to design, consume, and integrate with APIs.
In this context, we can also utilize this for GPT function calling by generating tools schema from this OAS file and implementing a wrapper function to send requests to API server. This implementation focuses on DigiTraffic with its OpenAPI specification file. Summary of the generation process:
Download OAS
Many OAS files are available or can be easily generated from an API server. We can download such files and open them as Python dictionary.
load_oas
load_oas (oas_url:str, destination:str, overwrite:bool=False)
Load OpenAPI Specification from URL or file.
Type | Default | Details | |
---|---|---|---|
oas_url | str | OpenAPI Specification URL | |
destination | str | Destination file | |
overwrite | bool | False | Overwrite existing file |
Returns | dict | OpenAPI Specification |
Load OAS from Road DigiTraffic:
= load_oas(
oas ="https://tie.digitraffic.fi/swagger/openapi.json",
oas_url="openapi.json",
destination=False
overwrite
)'paths'][list(oas['paths'].keys())[0]] oas[
{'get': {'tags': ['Beta'],
'summary': 'List the history of sensor values from the weather road station',
'operationId': 'weatherDataHistory',
'parameters': [{'name': 'stationId',
'in': 'path',
'description': 'Weather station id',
'required': True,
'schema': {'type': 'integer', 'format': 'int64'}},
{'name': 'from',
'in': 'query',
'description': 'Fetch history after given date time',
'required': False,
'schema': {'type': 'string', 'format': 'date-time'}},
{'name': 'to',
'in': 'query',
'description': 'Limit history to given date time',
'required': False,
'schema': {'type': 'string', 'format': 'date-time'}}],
'responses': {'200': {'description': 'Successful retrieval of weather station data',
'content': {'application/json;charset=UTF-8': {'schema': {'type': 'array',
'items': {'$ref': '#/components/schemas/WeatherSensorValueHistoryDto'}}}}},
'400': {'description': 'Invalid parameter(s)'}}}}
Deep reference extraction
In order to generate tool schemas, we need to resolve and flatten the references to components
. This process is complicated because of the nested references in components
, which may also include circular references and lead to efficiency issues.
To address these problems, the implemented algorithm involves:
- Traverse
components
and retrieve:
- List of all reference names in format
components/{section}/{item}
.
- Mapping of reference names to all locations where they are referenced by other references (nested reference). Locations are saved as strings in which each layer is separated by
/
.
- Mapping of reference names to all other references they refer to in their definitions (nested references) - dependencies mapping.
Check for “clean” references - references that do not include nested references in their definitions / references that do not have any dependencies.
Attach the dictionary definitions of the “clean” references to locations that they are referred to.
Update the dependencies mapping and “clean” references list. Repeat step 2, 3, and 4 until all references are “clean” or no more references can be “cleaned” (circular referencing).
Return a flat mapping of global reference names (formatted
#/components/{section}/{item}
) to their expanded (resolved) definitions.
"""
mm(flowchart TD
OC[OAS Components] -->|traverse| RL[Reference list]
OC -->|traverse| NRD[Nested reference dependencies]
OC -->|traverse| NRL[Nested reference locations]
NRD -->|check| CR[Clean references, i.e., no dependencies]
CR -->|compare| RL
RL -->|Clean < All| CR[Clean references]
subgraph Reference resolution
CR -->|attach| NRL
NRL -->|update| NRD
end
RL -->|Clean = All| EC[Flattened components]
RL -->|Clean unchanged| EC
""")
NOTE: For reference locations, layers being separated by /
may overlap with MIME types such as text/plain
and application/json
. Therefore, we need an utility function to scan for these MIME types and extract the correct layers:
retrieve_ref_parts
retrieve_ref_parts (refs:str)
Retrieve the parts of a reference string
Implementation of the described algorithm:
extract_refs
extract_refs (oas:dict)
Type | Details | |
---|---|---|
oas | dict | The OpenAPI schema |
Returns | dict | The extracted references (flattened) |
We can demonstrate an example usage with Road DigiTraffic. The API server includes a reference called Address
which involves nested referencing and circular referencing. The algorithm successfully expands possible parts of this reference and ignores the circular referencing.
= extract_refs(oas)
refs "#/components/schemas/Address"] refs[
{'type': 'object',
'properties': {'postcode': {'type': 'string'},
'city': {'required': ['values'],
'type': 'object',
'properties': {'values': {'required': ['values'],
'type': 'object',
'properties': {'values': {'type': 'array',
'xml': {'name': 'value',
'namespace': 'http://datex2.eu/schema/3/common'},
'items': {'type': 'object',
'properties': {'value': {'type': 'string'},
'lang': {'type': 'string', 'xml': {'attribute': True}}}}}}}}},
'countryCode': {'type': 'string'},
'addressLines': {'type': 'array',
'xml': {'name': 'addressLine'},
'items': {'$ref': '#/components/schemas/AddressLine'}},
'get_AddressExtension': {'$ref': '#/components/schemas/_ExtensionType'}}}
OAS schema to GPT-compatible schema
GPT currently recognizes only a limited number of descriptors when defining tools schema. Some of these descriptors (fields) can be directly transferred from OAS schema to tools, but many existing OAS schema fields will not be recognized by GPT and can cause errors. Therefore, transformation from OAS schemas to GPT-compatible schemas is necessary.
GPT currently recognizes these fields:
type
Specifies the data type of the value. Common types include:
string
– A text string.
number
– A numeric value (can be integer or floating point).
integer
– A whole number.
boolean
– A true/false value.
array
– A list of items (you can define the type of items in the array as well).
object
– A JSON object (with properties, which can be further defined with their own types).
null
– A special type to represent a null or absent value.
any
– Allows any type, typically used for flexible inputs or outputs.
default
: Provides a default value for the field if the user doesn’t supply one. It can be any valid type based on the expected schema.
enum
: Specifies a list of acceptable values for a field. It restricts the input to one of the predefined values in the array.
properties
: Used for objects, this defines the subfields of an object and their respective types.
items
: Defines the type of items in an array. For example, you can specify that an array contains only strings or integers.
minLength
,maxLength
: Specifies minimum and maximum lengths forstring
parameters.
minItems
,maxItems
: Specifies mininum and maximum number of items forarray
parameters.
pattern
: Specifies a regular expression that the string must match forstring
parameters.
required
: A list of required fields for anobject
. Specifies that certain fields within anobject
must be provided.
additionalProperties
: Specifies whether additional properties are allowed in anobject
. If set tofalse
, no properties outside of those defined in properties will be accepted.
As such, we can extract corresponding fields from OAS schema, and converts all additional fields into parameter description.
transform_property
transform_property (prop:dict, flatten_refs:dict={})
Type | Default | Details | |
---|---|---|---|
prop | dict | The property to transform | |
flatten_refs | dict | {} | The flattened references |
Returns | tuple | The transformed property and whether it is a required property |
Test usage with complex parameters from DigiTraffic endpoints:
= [
parameters
{"name": "lastUpdated",
"in": "query",
"description": "If parameter is given result will only contain update status.",
"required": False,
"schema": {
"type": "boolean",
"default": False
}
},
{"name": "roadNumber",
"in": "query",
"description": "Road number",
"required": False,
"schema": {
"type": "integer",
"format": "int32"
}
},
{"name": "xMin",
"in": "query",
"description": "Minimum x coordinate (longitude) Coordinates are in WGS84 format in decimal degrees. Values between 19.0 and 32.0.",
"required": False,
"schema": {
"maximum": 32,
"exclusiveMaximum": False,
"minimum": 19,
"exclusiveMinimum": False,
"type": "number",
"format": "double",
"default": 19
}
}
]
for param in parameters:
= transform_property(param)
param, required print(param)
print(f"Required: {required}\n")
print()
{'description': 'If parameter is given result will only contain update status. (Name: lastUpdated; In: query)', 'type': 'boolean', 'default': False}
Required: False
{'description': 'Road number (Name: roadNumber; In: query; Format: int32)', 'type': 'integer'}
Required: False
{'description': 'Minimum x coordinate (longitude) Coordinates are in WGS84 format in decimal degrees. Values between 19.0 and 32.0. (Name: xMin; In: query; Maximum: 32; Exclusivemaximum: False; Minimum: 19; Exclusiveminimum: False; Format: double)', 'type': 'number', 'default': 19}
Required: False
OAS to schema
We can combine the above utilities to extract important information about the functions and creates a GPT-compatible tools schema. The idea is to convert all necessary information for generating an API request to a parameter for GPT to provide. As such, the parameters of each function in this tools schema will include:
path
: dictionary for path parameters that maps parameter names to schemaquery
: dictionary for query parameters that maps parameter names to schemabody
: request body schema
Apart from the parameters that GPT should provide, we also need constant values for each function (endpoint). These values should be saved as metadata
in tools schema:
url
: URL to send requests to
method
: HTTP method for each endpoint
Auxiliary fixup function
To avoid writing Python functions for each of these endpoints, we can use a universal fixup (wrapper) function to execute API requests based on the above data.
NOTE: Apart from the above inputs, implementation of this fixup function will be more simple with an extra metadata
for acceptable query parameters. This is because GPT often flattens parameter inputs for simple requests, making it difficult to differentiate between path
and query
parameters.
generate_request
generate_request (function_name:str, url:str, method:str, path:dict={}, query:dict={}, body:dict={}, accepted_queries:list=[], **kwargs)
Generate a request from the function name and parameters.
Type | Default | Details | |
---|---|---|---|
function_name | str | The name of the function | |
url | str | The URL of the request | |
method | str | The method of the request | |
path | dict | {} | The path parameters of the request |
query | dict | {} | The query parameters of the request |
body | dict | {} | The body of the request |
accepted_queries | list | [] | The accepted queries of the request |
kwargs | VAR_KEYWORD | ||
Returns | dict | The response of the request |
API Schema
api_schema
api_schema (base_url:str, oas:dict, service_name:Optional[str]=None, fixup:Optional[Callable]=None)
Form the tools schema from the OpenAPI schema.
Type | Default | Details | |
---|---|---|---|
base_url | str | The base URL of the API | |
oas | dict | The OpenAPI schema | |
service_name | Optional | None | The name of the service |
fixup | Optional | None | Fixup function to execute a REST API request |
Returns | dict | The api schema |
Example with Road DigiTraffic:
= api_schema(
tools ="https://tie.digitraffic.fi",
base_url=oas,
oas="Traffic Information",
service_name=generate_request
fixup
)0] tools[
{'type': 'function',
'function': {'name': 'weatherDataHistory',
'description': 'List the history of sensor values from the weather road station',
'parameters': {'type': 'object',
'properties': {'path': {'type': 'object',
'properties': {'stationId': {'description': 'Weather station id (Name: stationId; In: path; Format: int64)',
'type': 'integer'}},
'required': ['stationId']},
'query': {'type': 'object',
'properties': {'from': {'description': 'Fetch history after given date time (Name: from; In: query; Format: date-time)',
'type': 'string'},
'to': {'description': 'Limit history to given date time (Name: to; In: query; Format: date-time)',
'type': 'string'}},
'required': []}},
'required': ['path']},
'metadata': {'url': 'https://tie.digitraffic.fi/api/beta/weather-history-data/{stationId}',
'method': 'get',
'accepted_queries': ['from', 'to'],
'service': 'Traffic Information'},
'fixup': '__main__.generate_request'}}
Simulated GPT workflow
Test integrating with our current GPT framework:
from llmcam.core.fc import *
= form_msgs([
messages "system", "You are a helpful system administrator. Use the supplied tools to help the user."),
("user", "Get the weather camera information for the stations with ID C01503 and C01504."),
(
])=tools)
complete(messages, tools print_msgs(messages)
>> System:
You are a helpful system administrator. Use the supplied tools to help the user.
>> User:
Get the weather camera information for the stations with ID C01503 and C01504.
>> Assistant:
Here is the weather camera information for the stations with ID C01503 and C01504: ### Station
C01503 - **Name**: Road 51 Inkoo (Tie 51 Inkoo in Finnish) - **Location**: Municipality of Inkoo in
Uusimaa province - **Coordinates**: Latitude 60.05374, Longitude 23.99616 - **Camera Type**: BOSCH -
**Nearest Weather Station ID**: 1013 - **Collection Status**: Gathering - **Updated Time**:
2024-12-10T15:25:40Z - **Collection Interval**: Every 600 seconds - **Preset Images**: -
Inkooseen: [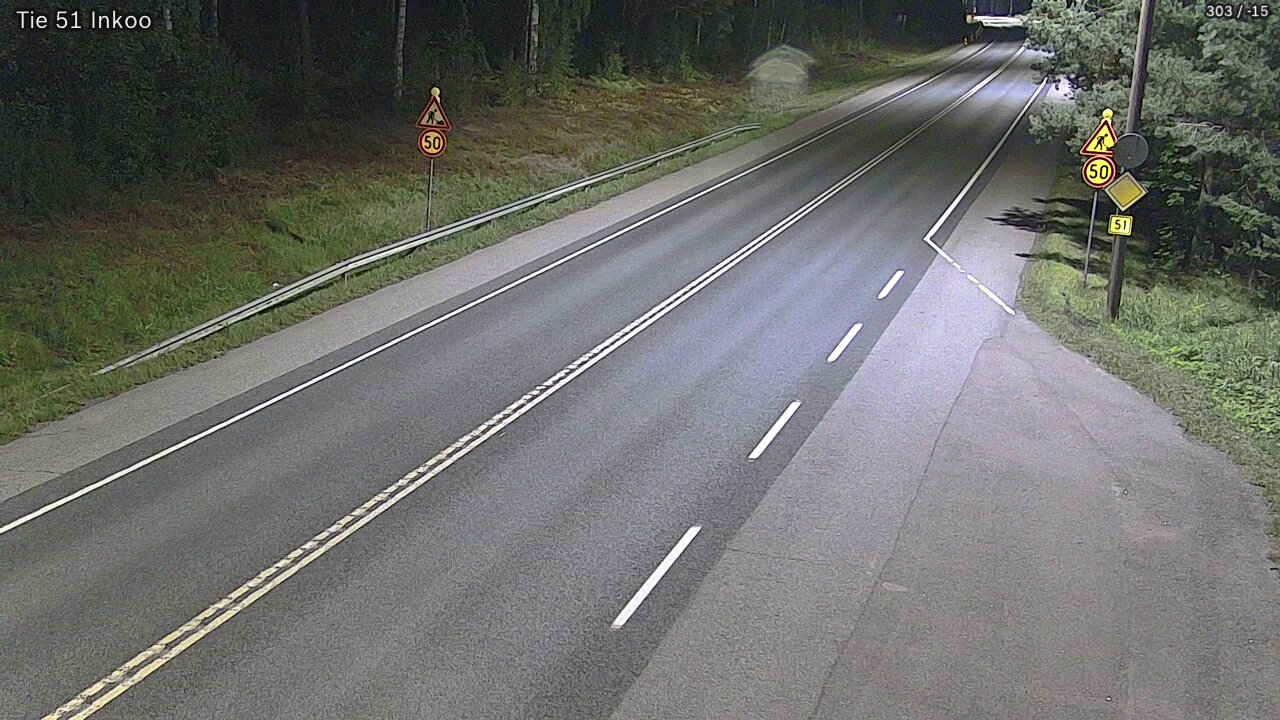](https://weathercam.digitraffic
.fi/C0150301.jpg) - Hankoon: [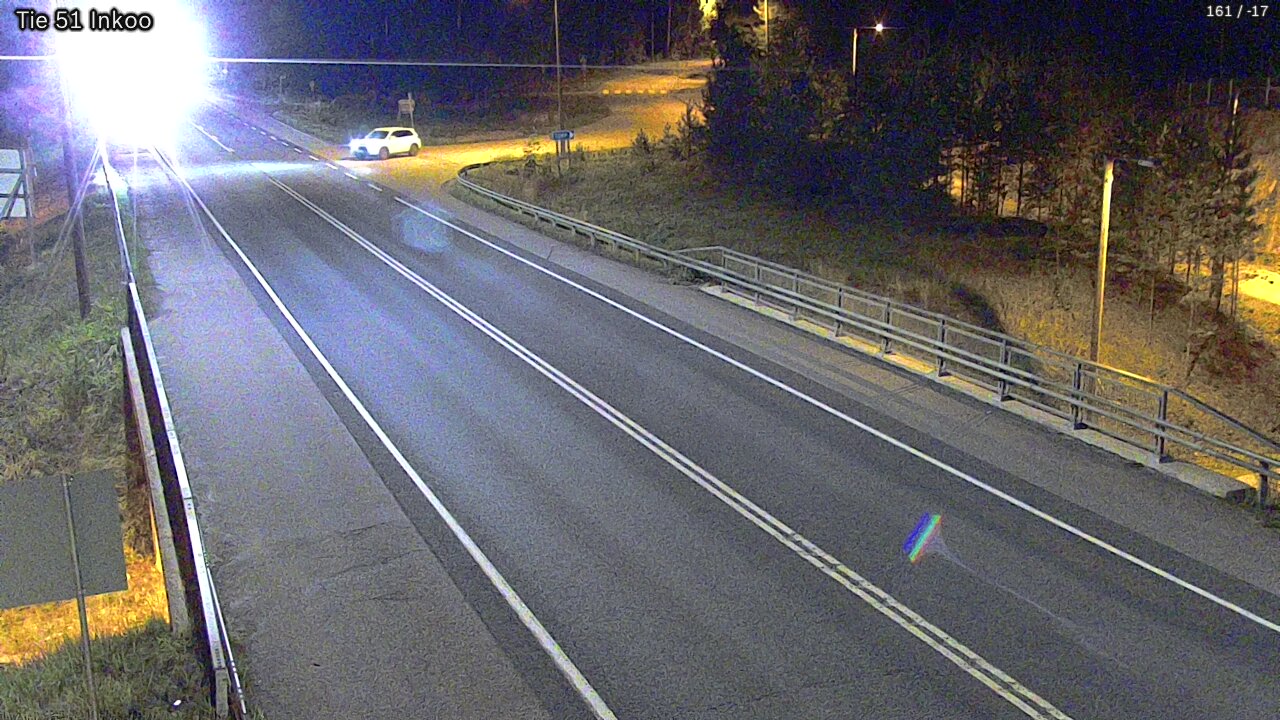](https://we
athercam.digitraffic.fi/C0150302.jpg) - Tienpinta: [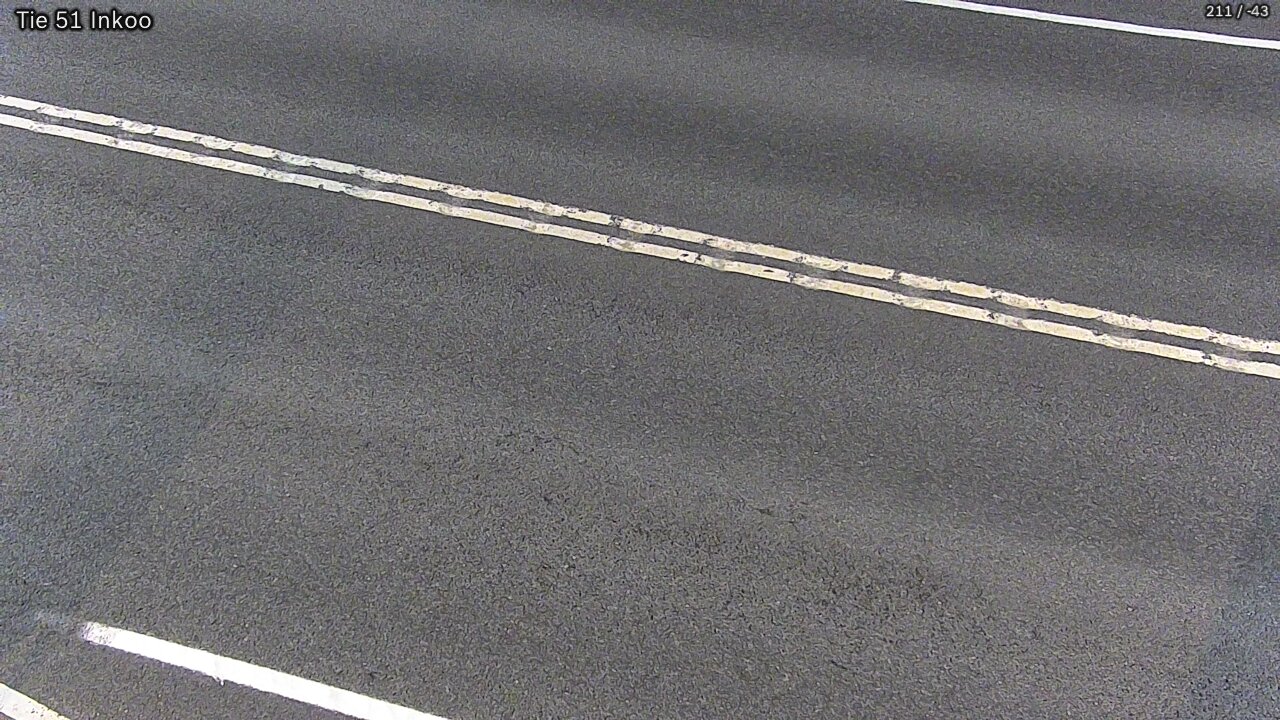](https://weathercam.digitraffic.fi/C0150309.jpg) ### Station C01504 - **Name**: Road 2
Karkkila, Kappeli (Tie 2 Karkkila, Kappeli in Finnish) - **Location**: Municipality of Karkkila in
Uusimaa province - **Coordinates**: Latitude 60.536727, Longitude 24.235601 - **Camera Type**:
HIKVISION - **Nearest Weather Station ID**: 1052 - **Collection Status**: Gathering - **Updated
Time**: 2024-12-10T15:27:01Z - **Collection Interval**: Every 600 seconds - **Preset Images**: -
Poriin: [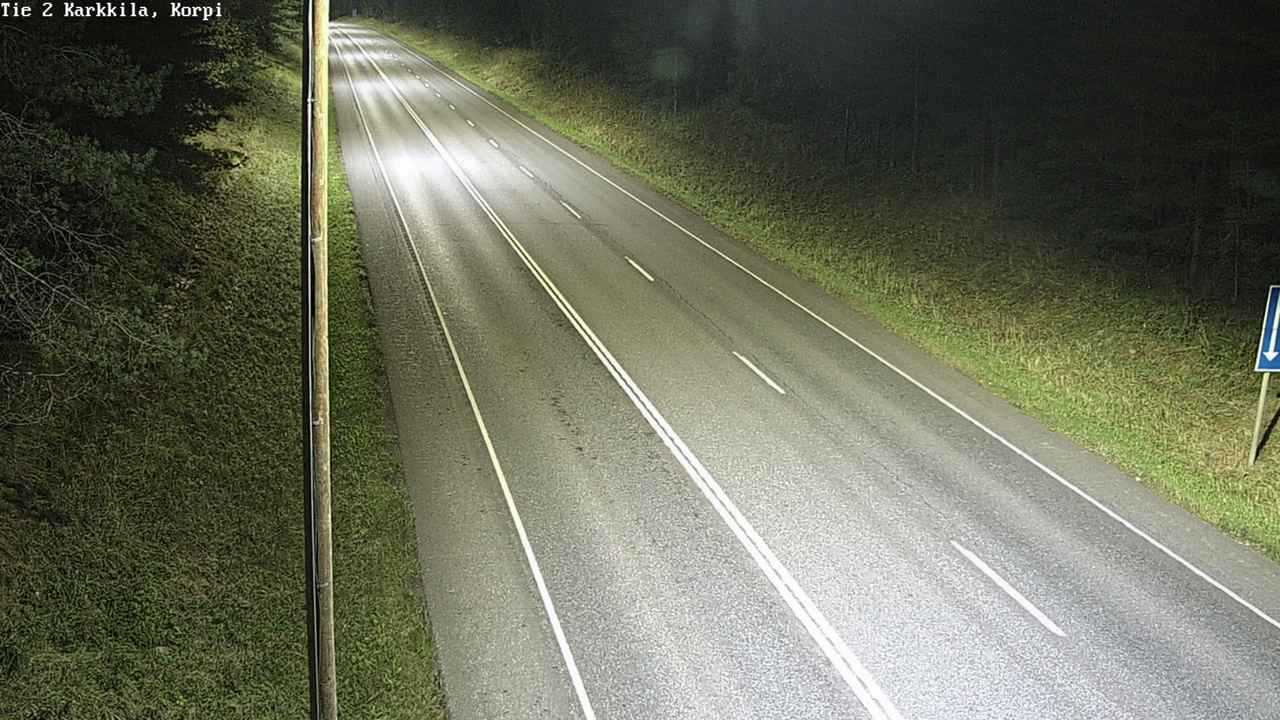](https://weathercam.digitraffic.fi
/C0150401.jpg) - Helsinkiin: [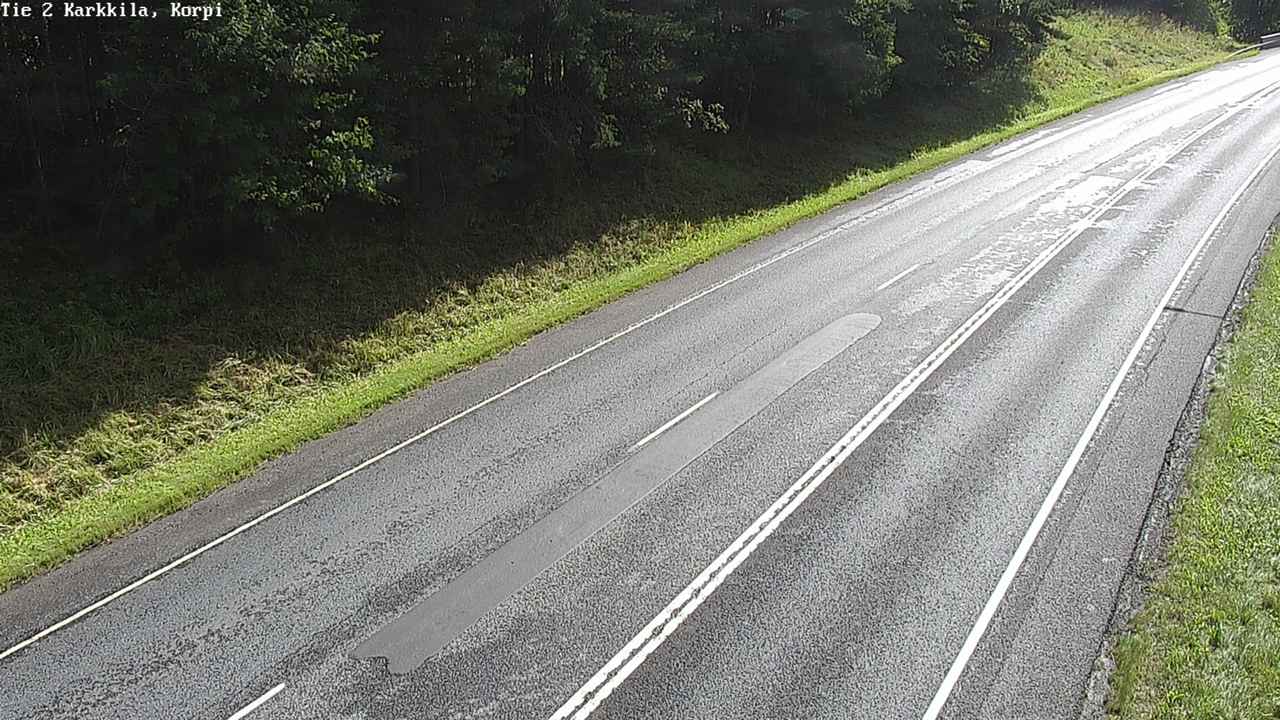](https://we
athercam.digitraffic.fi/C0150402.jpg) - Tienpinta: [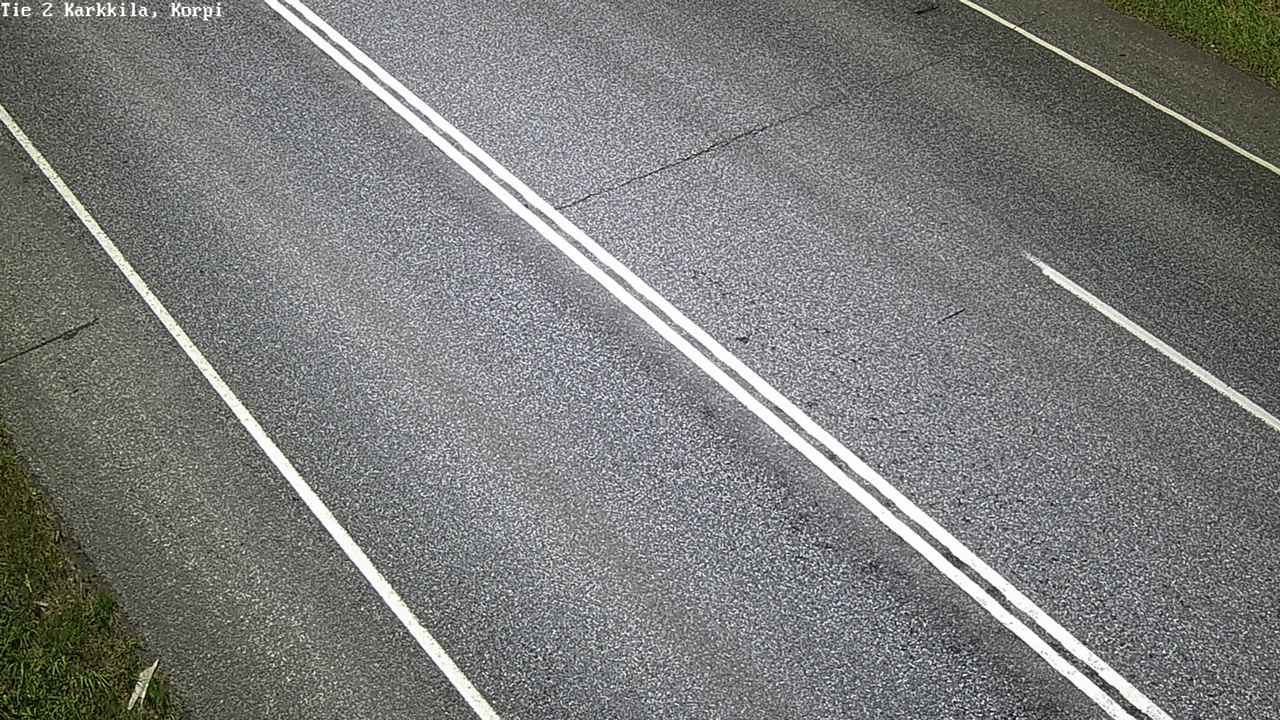](https://weathercam.digitraffic.fi/C0150409.jpg) If you have any more questions or need
further assistance, feel free to ask!